American political-party affiliation as a predictor of usage of an adultery website
Kodi B. Arfer and Jason J. Jones
Created 11 May 2016 • Last modified 30 May 2018
The more politically conservative Americans are, the more restrictive their sexual attitudes are. A natural follow-up question is how this difference in attitudes relates to actual behavior. But self-reports of sexual behavior may be compromised by a social desirability bias that is influenced by the very sexual attitudes at issue. We employed a non-self-reported measure of sexual behavior: usage of the adultery-focused dating website Ashley Madison. Linking an August 2015 leak of user data from Ashley Madison to 2012 voter registration rolls from five US states, we found 80,000 matches between 200,000 Ashley Madison user accounts and 50 million voters. According to simple rates in the sample, and also to predictively validated regression models controlling for state, gender, and age, we found that Democrats were least likely to use Ashley Madison, Libertarians were most likely, and Republicans, Greens, and unaffiliated voters were in between. Our results provide support for theories arguing that people with stricter sexual attitudes are paradoxically more likely to engage in deviant sexual behavior.
This paper was published as:
Arfer, K. B., & Jones, J. J. (2019). American political-party affiliation as a predictor of usage of an adultery website. Archives of Sexual Behavior, 48, 715–723. doi:10.1007/s10508-018-1244-1
The official Springer PDF has only minor differences from this the original document.
Keywords: adultery, online dating, political-party affiliation, Democratic Party, Republican Party
Acknowledgments: This project was supported by the National Institute of Mental Health (T32MH109205).
Conflicts of interest: Both authors are registered to vote as Democrats. The authors declare that they have no other potential conflicts of interest.
Introduction
American political conservatism emphasizes maintenance of the social order, individual interests (as opposed to collective interests), limited government, aggressive foreign policy, and traditional or religious values. The American political arena is dominated by two major parties, Republican and Democratic, with the Republican Party being more conservative. In general, more politically conservative Americans are also more sexually conservative, in both the attitudes they espouse and the behavior they self-report. Fried (2008), comparing Republicans and Democrats in General Social Survey (GSS) data, found that Democrats saw premarital sex as more acceptable, had had more sex partners, and were more likely to have had a same-sex partner or participated in prostitution (whether buying or selling). Gallup (2003) found similarly that 80% of liberals but only 42% of conservatives saw premarital sex as acceptable. A meta-analysis by Whitley and Lee (2000) found that conservative views, as well as the related personality constructs of authoritarianism and social-dominance orientation, were associated with more negative attitudes towards homosexuality. Since more religious people (especially conservative Protestants) are more likely to be Republicans than Democrats (Pew Research Center, 2015), and religions (especially conservative Protestantism) tend to promote sexual conservatism, it is no surprise that more religious and more religiously conservative Americans tend to also have more negative views of premarital sex (Petersen & Donnenwerth, 1997), homosexuality (Hill, Moulton, & Burdette, 2004), and pornography (Carroll et al., 2008).
One might wonder how much conservatives' actual sexual behavior is in line with their attitudes, and how honest they are in reporting their behavior. Sex can be tempting, and people with stricter sexual standards should be all the less willing to admit their indiscretions to prying researchers. Some writers, inspired by sex scandals involving conservative public figures such as Republican senator Larry Craig (McArdle, 2007) and commentator Bill O'Reilly (Steel & Schmidt, 2017), have speculated that more sexually conservative people are paradoxically more sexually deviant in practice. Some support for this idea comes from two state-level analyses. Edelman (2009) found that states with more conservative attitudes towards religion, sexuality, and marriage buy more subscriptions per capita to pornographic websites. MacInnis and Hodson (2015) found that more religious states had more Google searches for the word "sex," and more politically conservative states had more Google Images searches for "sex."
Why might stricter attitudes be associated with less restricted behavior? Freud (1910) popularized the idea that when people refuse to act on sexual urges, possibly unconscious ones, out of ethical concerns or other restraints of civilization, they may "flee to sickness, in order to find with its help a surrogate satisfaction" (p. 213). According to Freud, the "surrogate satisfaction" could be neurotic behavior with no apparent sexual significance, but it could also be sexual "perversion." Freud also argued for a hydraulic model of male sexuality in which going without ejaculation created "pressure on the walls of the seminal vesicles" (quoted in Apfelbaum, 1984). However, empirical evidence that unexpressed sexual desire builds up over time is weak at best (see Arfer, 2016, for a review). To return to conservatives in particular, some investigators have even taken sexual repression (Sidanius, 1985) or sexual preoccupation (Adorno, Frenkel-Brunswik, Levinson, & Sanford, 1950) to be a defining feature of the right-wing personality.
Even when simple self-report of sexual behavior is examined, we find evidence that people fall short of their own standards. In a sample of urban adolescents, Zabin, Hirsch, Smith, and Hardy (1984) found that 83% of those who had had sex endorsed a best age of sexual debut that was older than their own age of sexual debut. Similarly, 88% of the women who had had a child endorsed a best age for one's first pregnancy that was older than their own age of first pregnancy. In college students who had had sex or petted outside a concurrent committed relationship, Feldman and Cauffman (1999) found the mean acceptance of such activity to be 1.86 on a scale from 1 (totally unacceptable) to 4 (totally acceptable). At the same time, both these studies found positive relationships between people's acceptance of such behavior and their reports of committing it.
By discussing discrepancies between attitudes and self-reported behavior, we do not mean to imply that people are always honest about their behavior. On the contrary, studies have found that the bogus-pipeline method, in which people are falsely told they are subject to some kind of lie-detecting device, can change the rate at which people report deviant behavior. Alexander and Fisher (2003) found that a bogus pipeline increased women's odds of reporting masturbation and pornography consumption. Strang and Peterson (2016) found that a bogus pipeline increased men's odds of reporting sexual assault. Oddly, Fisher and Brunell (2014) found that a bogus pipeline decreased men's odds of reporting extradyadic sexual activity. Overall, while Zabin et al. (1984) and Feldman and Cauffman (1999) point to a gap between attitudes and behavior, and bogus-pipeline studies show that self-report may not be honest, neither kind of study offers a full alternative to self-report.
In this study, we will focus on sexual infidelity, particularly adultery, which is reasonably common (17% of GSS respondents said they had had extramarital sex; Burdette, Ellison, Sherkat, & Gore, 2007) but widely frowned upon regardless of political orientation (a survey of 1,005 people found that 6% of conservatives and 9% of liberals deemed it acceptable; Gallup, 2003). More religious and more religiously conservative people are less likely to state that they or their spouse have had extramarital sex (Burdette et al., 2007; Tuttle & Davis, 2015; but contrast Atkins, Baucom, & Jacobson, 2001), as do Republicans compared to Democrats (Fried, 2008), and more politically conservative people estimate adultery in their marriages as less likely (Buss & Shackelford, 1997).
Our study obtained an objective, individual-level measure of adultery (more precisely, intention to cheat in one's own or another's committed relationship) by drawing on data from the 2015 leak (Victor, 2015) of Ashley Madison (AM; http://www.ashleymadison.com), a dating website that specializes in cheating (slogan until 2016: "Life is short. Have an affair."). Previous uses of this data in social science include Grieser, Kapadia, Li, and Simonov (2016) and Griffin, Kruger, and Maturana (2016). We linked AM users with voter registration records to determine how usage of AM could be predicted on the basis of political-party affiliation, gender, age, and state.
Method
Subjects
All data-processing and analysis code can be found at http://arfer.net/projects/cheat. We used two sources of data: voter registration rolls from five US states (California, Florida, Kansas, New York, and Oklahoma) in 2012, and records of AM users from the 2015 leak by a group calling itself the Impact Team. To determine whether each voter had used AM, we attempted to find a matching AM credit-card payment.
Measures and Procedure
Processing the voter registration rolls
Regarding voter registration rolls, we chose states on the basis of data that were readily available and out of a desire to cover a variety of geopolitical groups in the US. We selected all voters in the data for whom the registration record was well-formed (e.g., had a field for each column) and at least one valid value for each of the four kinds of data items used for matching: first names, last names, 5-digit ZIP codes, and address numbers. (We used just the numeric portion of addresses so we did not have to manage issues of spelling and abbreviations.) We saved one of each of these kinds, except in the case of address numbers, of which we saved up to two. ZIP codes that did not lie in the state from which the voting records came (according to the database http://federalgovernmentzipcodes.us/free-zipcode-database-Primary.csv, which draws from United States Postal Service data) were considered invalid. When there were multiple records for the same numeric voter ID in the same state, we used only the one that appeared first in the voter data. Overall, we saved records for 48,852,975 voters. Table 1 compares the population of each state to the number of voter records we obtained.
State | California | Florida | Kansas | New York | Oklahoma |
---|---|---|---|---|---|
Unaffiliated voters | 2,710,719 | 2,700,397 | 522,094 | 3,016,645 | 229,494 |
Democrats | 7,936,325 | 4,988,433 | 432,858 | 7,134,846 | 879,343 |
Republicans | 5,297,443 | 4,378,861 | 773,346 | 3,484,717 | 844,305 |
Greens | 114,126 | 6,143 | 0 | 32,811 | 0 |
Libertarians | 110,808 | 12,016 | 11,690 | 4,710 | 0 |
Voters in other party | 2,004,915 | 429,399 | 0 | 796,528 | 3 |
Total registered voters | 18,174,336 | 12,515,249 | 1,739,988 | 14,470,257 | 1,953,145 |
Population | 38,041,430 | 19,317,568 | 2,885,905 | 19,570,261 | 3,814,820 |
Percent covered | 48 | 65 | 60 | 74 | 51 |
AM users matched with voters | 32,457 | 17,928 | 2,642 | 21,776 | 2,072 |
Total AM users | 92,058 | 43,987 | 6,282 | 54,203 | 6,603 |
Percent matched | 35 | 41 | 42 | 40 | 31 |
In addition to this basic matching information, we saved each voter's political party, gender, date of birth, and date of registration. Not all of this information was available for all voters. Gender in particular was often missing (the Oklahoma records had no gender information at all), so we imputed it using known genders elsewhere in the voting data (for any state) on the basis of first name: when a large majority of people with known gender and the same first name were male or female, the missing gender was imputed to be the same. (Imputing gender using first names appears in, for example, Blevins & Mullen, 2015; Yoon, Tourassi, & Xu, 2015. Our specific method is that when p ≤ .05 or p ≥ .95, where p = (f + 1)/(n + 2), f is the number of females with the name, and n is the number of known-gender voters with the name (so p is the posterior mean of a Bernoulli distribution with a flat prior), we imputed the gender as male or female, respectively.) Overall, 18% of genders were missing, and we imputed 87% of these missing genders, leaving the final missing rate at 2.3%.
When date of birth was missing, we did not impute it. However, we discarded some dates of birth which were present but seemed unlikely to be correct. These included dates of birth on or after the date of registration; dates in the 21st century; impossible dates, such as September 31st or dates with month 0; and dates with unrealistically high concentrations of voters, such as 10 October 1942 in the Florida records. Across states, 1 in 68,000 birthdates were initially missing, but after discarding, 1 in 95 were missing.
A complexity of party affiliation arises from confusion about the term "independent." California, Florida, and New York have large numbers of voters (479,378, 339,002, and 557,885, respectively) registered to a party called an "Independent Party" or "Independence Party." These voters may have intended to indicate that they wished to be independent; that is, to not belong to any party. Independence Party of New York (2014) states "The Party's leadership recognizes that individuals do sometimes unwittingly register as members of the Independence Party when their intent was to register to vote as a 'blank.'" Because there is no way to determine which of these voters meant to register as unaffiliated, we treated them all as part of the "other party" group, which is not included in the analyses of parties.
Processing the AM data
We used three data files from the AM leak. From CreditCardTransactions.7z
, we obtained names, addresses, and ZIP codes for each credit-card payment on AM from 21 March 2008 to 28 June 2015. We selected only successful payment transactions with an Address Verification System code that indicated both the address and the ZIP code had matched against what was on record for the card. (Address Verification Systems do not check personal names in most cases.) We obtained 2,344,452 payments with US ZIP codes,
which involved a total of 738,674 AM users,
203,133 of whom had ZIP codes for states in the voter data.
From am_am.dump.gz
and aminno_member.dump.gz
, we obtained a list of AM users who had paid to delete their account. AM did not actually delete most of the information of each user who paid for such deletion, which was among the kinds of wrongdoing charged by the Federal Trade Commission for which an $18 million settlement was obtained from AM's owner, Avid Life Media, now called Ruby Corp. (Geuss, 2016).
A caveat of using AM's credit-card transaction data is that female users of AM weren't charged some of the fees that male users were (Lamont, 2016). Hence, our analyses will miss an unknown proportion of female users. From user-entered demographic data in am_am.dump.gz
, we see that indeed only a small minority (2.4%) of the collected AM users were female. Race was also lopsided, with 81% of users identifying as White, 6.1% as Hispanic, 5.5% as Black,
5.8% as Other,
and 1.7% not responding
(users could only choose one option). We consulted a source-code file from the AM leak, AMLIB_SelectOptions.class.php
, to interpret numeric race codes. Finally, user-entered dates of birth imply that the median age in 2012 was 41 years and the middle 95% of users were 23 to 62 years old in 2012.
Matching
The matching algorithm worked by taking each user who made an AM credit-card payment and searching for a voter with the same first name, last name, and ZIP code, and at least one equal address number. If more than one voter satisfied these criteria for a given payment, none of the voters were treated as matching the AM user. On the other hand, if more than one AM user satisfied this criterion for a single voter, we treated this the same in our analyses as a unique match, since our only concern in the analyses was whether a voter used AM, not which AM account they used. We matched 78,296 voters to at least one AM user.
The analyses treated each voter as a subject, voter characteristics as independent variables, and whether the voter used AM as a dichotomous dependent variable. We assumed that matching a credit-card transaction, as described above, was a good measure of whether a voter made a serious attempt to cheat via AM; by not treating people who never paid money as AM users, we distinguish people who created an account but never attempted to cheat. An exceptional case was users who only paid to delete their account. These users may well have had an account created for them by somebody else to discredit them and, in any case, did not pay to cheat. Hence, we treated any matching voter identified by the above procedure as not using AM if they had exactly one credit-card payment and they paid to delete their account, which implies that the payment was for deletion. Among matching voters, 1,421 were treated as nonusers of AM for this reason, for an effective total AM usage rate of 76,875 out of 48,852,975 voters (1 in 635), several orders of magnitude below the self-reported adultery rate of 17% in the GSS (Burdette et al., 2007). Table 1 compares the number of AM users in each state to the number matched up with voters.
Results
We present two basic analytic approaches: a simple approach that groups the sample into bins and counts frequencies, and a more complex approach based on logistic regression and cross-validation. Our analyses included subjects belonging to no party as well as to any of four parties, namely, the two major American parties, Democratic and Republican, plus two of the largest minor parties, Green and Libertarian. In our informal judgment, Greens are less conservative than Democrats, whereas Libertarians are harder to rate in terms of overall conservatism, but on average are more conservative than Republicans. Note that as shown in Table 1, not every state listed any voters registered as Greens or Libertarians.
In the simple approach, we counted how many voters used AM within each state and party. The results are shown in Figure 1. We see that across all five states, Democrats had the least AM usage of all party groups, and in the four states where Libertarians are present, they used AM the most, with a rate of at least 1 in 300 in each state. Republicans and unaffiliated voters had intermediate rates that were similar to each other. Greens used AM more than Republicans in California and Florida, but less in New York. As for effects of state alone, ignoring parties (and hence including voters in parties other than these four), California had the most AM usage (1 in 560 voters), Oklahoma had the least (1 in 943), and the remainder were intermediate (1 in 659 for Kansas, 1 in 665 for New York, and 1 in 698 for Florida).
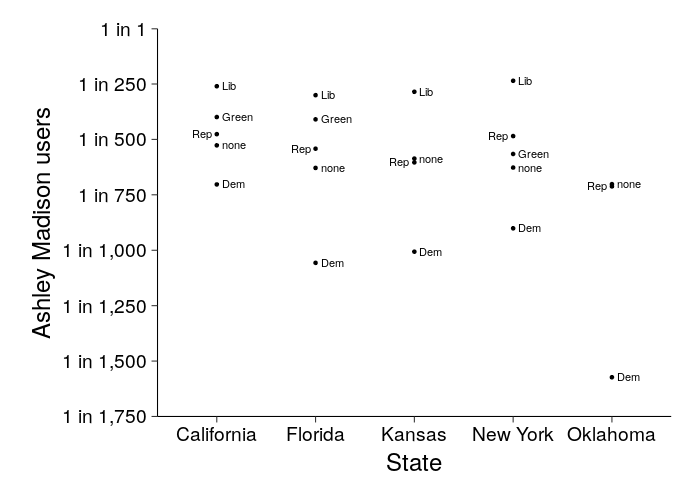
The analysis represented by Figure 1 is easy to understand, but crude, because it does not account for, for example, gender differences in party affiliation. In our data, 63% of Libertarians but only 42% of Democrats were male, and 1 in 301 men but only 1 in 8,686 women used AM, so it is worth distinguishing the effect of party from the effect of gender.
To estimate the predictive value of party above and beyond confounding variables such as age and gender, we investigated a family of logistic regression models, each regularized with an L2 (ridge) penalty. Logistic regression is an appropriate technique for modeling the probabilities of binary events. Regularization, also known as shrinkage methods, improves predictive accuracy by biasing regression coefficients towards 0 and hence countering overfitting, the tendency of statistical models to mistake noise for signal. Our models treated each voter as a Bernoulli trial (that is, a 0-or-1 binary event) with the outcome being whether the voter used AM. The models used state, gender, age, and party affiliation as predictors. Subjects with unimputed genders, missing ages, or parties other than the four analytic parties were removed, leaving a sample of 44,172,769 voters, 69,023 of which (1 in 640) used AM. State and party affiliation were dummy-coded, with New York and unaffiliated voters as the respective reference groups. Age was coded as the difference between the voter's year of birth and 2012, then standardized to have mean 0 and SD 0.5 (Gelman, 2008), and supplemented with a similarly standardized age-squared term.
We considered six models, which were distinguished by the choice of predictors, drawing from among state, gender, age, and party. All nontrivial models were fit with Python 3.6 and Hy 4fce884, using the LogisticRegression
class from scikit-learn 0.18.1. We used tenfold cross-validation to estimate the predictive accuracy of each model, randomly splitting the voter data into ten folds and then, for each fold in turn, fitting model parameters using the other nine folds and having the model predict the probability of using AM for each voter in the held-out fold. The L2 penalty size C was chosen among {10−12, 10−11, …, 1011, 1012} using inner rounds of fivefold cross-validation. We compared predicted probabilities to actual probabilities with mean squared error (MSE), also known in the context of probabilistic classification as the Brier score, which is a proper scoring rule (Brier, 1950; Bröcker, 2009).
Descriptions of each model and cross-validation results are shown in Table 2. By MSE, the models from least to most accurate were Trivial, Parties, Demo, IntDemo, DemoParties, IntDemoParties. Hence, not only does political party have predictive value on its own; it adds to the predictive ability of a model already accounting for age, gender, and state. Examining p0 and p1 helps to get a more concrete idea of this predictive performance. p0 is the mean predicted probability of AM usage among voters who did not actually use AM, so that a p0 of 1 in 500 would mean that the model predicted on average that nonusers had a 1 in 500 chance of using AM. p1 is the same value among voters who did use AM. Thus, more accurate models have smaller p0s but larger p1s. We see that p0 does not improve much in any case: the models were generally unable to identify nonusers as less likely to use AM than the base rate. However, p1 improved substantially as predictors were added to the model, and interaction terms also improve p1, although only slightly. We will emphasize DemoParties in interpretation, since it is almost as accurate as IntDemoParties while being substantially simpler, and our interest in this study was more in the overall effects of party affiliation than interactions of party affiliation with state, age, or gender.
Model | Description | Terms | MSE | p0 | p1 |
---|---|---|---|---|---|
Trivial | No predictors | 1 | 0.001560127 | 1 in 640 | 1 in 640 |
Parties | Predictors: party only | 5 | 0.001559982 | 1 in 640 | 1 in 604 |
Demo | Predictors: state, gender, age | 8 | 0.001555791 | 1 in 642 | 1 in 232 |
DemoParties | Predictors: state, gender, age, party | 12 | 0.001555600 | 1 in 642 | 1 in 226 |
IntDemo | Demo, plus all first-order interactions | 22 | 0.001555783 | 1 in 642 | 1 in 231 |
IntDemoParties | DemoParties, plus all first-order interactions | 51 | 0.001555565 | 1 in 642 | 1 in 224 |
Having validated DemoParties and IntDemoParties as predictively accurate, we fit each model to all of the data (again using fivefold cross-validation to choose C) and examined the coefficients, which are shown in Table 3. We see that women were especially unlikely to use AM (since the coefficients are in logit units, the value −3.31 indicates that a male voter with a 50% chance of using AM would have his probability reduced to 3.5% if he were female); recall, however, that this effect is likely to have been inflated by women's underrepresentation in the AM credit-card data. In DemoParties, the terms for age describe a positive effect on AM usage from ages 25 to 63, peaking at age 44 with an effect of +1.04. Compared to New York, all states except California were associated with less AM use (except that IntDemoParties gives Florida a very small positive effect). In order from least to most AM-using, the parties were Democratic, unaffiliated, Green, Republican, and Libertarian. This agrees with the simple analysis of Figure 1, with the possible exception of the position of the Greens, which was also inconsistent between DemoParties and IntDemoParties.
Term | DemoParties | IntDemoParties |
---|---|---|
Intercept | −6.20 | −6.22 |
Age | −1.07 | −1.10 |
Age² | −2.23 | −2.14 |
Female | −3.31 | −3.13 |
Female × Age | 0.52 | |
Female × Age² | 0.43 | |
Female × Party: Democratic | 0.18 | |
Female × Party: Green | −0.08 | |
Female × Party: Libertarian | 0.03 | |
Female × Party: Republican | −0.01 | |
Female × State: California | −0.14 | |
Female × State: Florida | −0.14 | |
Female × State: Kansas | −0.23 | |
Female × State: Oklahoma | −0.23 | |
Party: Democratic | −0.18 | −0.17 |
Party: Democratic × Age | −0.13 | |
Party: Democratic × Age² | 0.08 | |
Party: Green | 0.02 | −0.19 |
Party: Green × Age | 0.22 | |
Party: Green × Age² | −0.29 | |
Party: Libertarian | 0.46 | 0.47 |
Party: Libertarian × Age | −0.34 | |
Party: Libertarian × Age² | −0.34 | |
Party: Republican | 0.19 | 0.28 |
Party: Republican × Age | −0.21 | |
Party: Republican × Age² | 0.05 | |
State: California | 0.14 | 0.16 |
State: California × Age | 0.22 | |
State: California × Age² | −0.19 | |
State: California × Party: Democratic | 0.06 | |
State: California × Party: Green | 0.23 | |
State: California × Party: Libertarian | −0.15 | |
State: California × Party: Republican | −0.16 | |
State: Florida | −0.04 | 0.01 |
State: Florida × Age | 0.19 | |
State: Florida × Age² | −0.22 | |
State: Florida × Party: Democratic | −0.13 | |
State: Florida × Party: Green | 0.42 | |
State: Florida × Party: Libertarian | −0.25 | |
State: Florida × Party: Republican | −0.10 | |
State: Kansas | −0.10 | −0.16 |
State: Kansas × Age | −0.33 | |
State: Kansas × Age² | −0.59 | |
State: Kansas × Party: Democratic | −0.07 | |
State: Kansas × Party: Libertarian | −0.08 | |
State: Kansas × Party: Republican | −0.25 | |
State: Oklahoma | −0.31 | −0.15 |
State: Oklahoma × Age | 0.23 | |
State: Oklahoma × Age² | −0.14 | |
State: Oklahoma × Party: Democratic | −0.26 | |
State: Oklahoma × Party: Republican | −0.20 |
Table 4 provides an example to make the models' predictions concrete. The order of parties was the same between the two models except for, again, the position of Greens. We see that while all men of this age in New York were expected to have a higher rate of AM usage than the base rate (1 in 640), party affiliation can still make a big difference.
Party | DemoParties | IntDemoParties |
---|---|---|
Libertarian | 1 in 117 | 1 in 98 |
Republican | 1 in 152 | 1 in 138 |
Green | 1 in 180 | 1 in 219 |
Unaffiliated | 1 in 184 | 1 in 189 |
Democratic | 1 in 219 | 1 in 223 |
Discussion
Using two analytic strategies, one simple and one complex, we found that a registered voter's probability of spending money on AM for something other than deleting their account—hence, apparently intending to cheat in a romantic relationship—varied substantially based on their political party. Libertarians were most likely to use AM, Democrats were least likely, and Republicans, Greens, and unaffiliated voters were in between. This pattern mostly coincides with political conservatism, with members of more conservative or more right-wing parties using AM more often.
Implications
Our results are perhaps the strongest evidence yet that people with more sexually conservative values, although they claim to act accordingly, are more sexually deviant in practice than their more sexually liberal peers. If this is true, what could explain it? We speculate that the explanation lies not with attempting sexual self-control in the first place, but with the other attendant circumstances of sexual conservatism, such as reduced knowledge of sexuality (Coleman & Testa, 2008) due to weak or nonexistent formal sex education (Kirby, 2007; Stanger-Hall & Hall, 2011) and less forthright discussion of sexual matters. It would make sense if less sexually knowledgeable people were worse at sexual self-control. More religious people may also have difficulty with sexual self-control if they attempt to rely on supernatural help to restrain their impulses. Perhaps expanding sex education and weakening taboos against the mere discussion of sex are ways by which society could reduce the incidence of adultery.
Another possibility is that many people endorse conservative sexual attitudes strategically, rather than out of earnest belief. They are interested in taboo sexual behavior, such as adultery, but wish to hide it. To deflect suspicion, they claim to be particularly committed to sexual restraint. Whether such a strategy is effective—that is, whether the general public believes these claims—is an open question. A weakness of this explanation is that it would be very difficult to test. The usual gold standard for a person's beliefs is their own statements, so it would be difficult to catch a person lying about their own beliefs.
While researchers are rarely so naive as to assume that people's behavior is perfectly concordant with their attitudes, it would seem obvious that attitudes and behavior should be positively, not negatively, related. This study, as well as Edelman (2009) and MacInnis and Hodson (2015), indicate there is, in fact, a negative relation, at least when it comes to political beliefs and sexual indulgence. It is thus possible that the positive relationships between permissive sexual attitudes and sexual activity observed by Zabin et al. (1984) and Feldman and Cauffman (1999) are artifacts of the use of self-report. After all, people with stricter sexual attitudes have all the more motivation to underreport their sexual behavior.
Limitations
Our study had two notable strengths in its very large sample and its avoidance of self-report and informant report. On the other hand, many limitations apply to its conclusions. First, the algorithm we used to match AM users and voters cannot be perfectly accurate, and we do not know how accurate it is. It is at least strict enough that misses are likelier than false positives.
Another issue is that while we would have liked to capture all cheating events, our data can only distinguish between users and nonusers of AM. There is no way to tell how much of the variation we observed was due to actual variation in cheating, and how much was due to variation in means of obtaining people to cheat with; comparing the GSS to our data implies that only a tiny minority of cheating events are aided with AM. The relationship between party and cheating by any means may be totally different from the relationship between party and AM use. For example, perhaps Democrats cheat more than Libertarians, but they can more easily find partners in person and hence have less need for AM to accomplish this.
Furthermore, party affiliation is contaminated with some other variables we would have liked to control for but we lacked data for, such as marriage. Married people are probably more likely to use AM, and Republicans are more likely to be married than Democrats (Fried, 2008). Controlling for age does at least help ameliorate our inability to control for marriage. Another variable of interest is income: AM costs money, at least for men, so wealthier men may use AM more, and Republicans are wealthier than Democrats (Pew Research Center, 2009). Here we do not have an appropriate substitute. Future research could approximate control variables with geographic aggregates, such as the median income for the ZIP code of each voter. While likely worth undertaking, such an analysis would unfortunately forgo the present study's strength of using fully individual-level data. An inverse concern is that party affiliation is only an imperfect measure of sexual attitudes. Finally, the lack of existing research on political attitudes, sexual attitudes, and self-reported sexual behavior among Libertarians and Greens hinders the interpretation of our results for them.
Ethical Considerations
Using data from the 2016 AM leak for scientific research raises ethical questions. The Impact Team's original act of obtaining and publishing the data would generally be regarded as unethical, for reasons ranging from illegally accessing AM's private files to compromising the privacy of AM's many users. Does it follow that using the published data is inherently unethical, even when following the usual guidelines for ethical research? An analogous issue exists for the more serious case of the murderous Nazi research on hypothermia: the original experiments should not have been conducted, so should we refrain from citing the experimenters' reports (Moe, 1984)? We believe that using data that was originally collected unethically is itself ethically permissible. To forbid such use would be closing the stable door after the horse has bolted. In the case of AM in particular, not only have the data already been publicly available since 2015, it has been widely discussed in the news (e.g., Biddle, 2015; Lamont, 2016; Victor, 2015), with some reports even describing how to obtain and use the data (e.g., Paton, 2015; Prince, 2015). We cannot undo the past, but we can make the most of the present by getting what social and scientific value we can out of undesirable events, whether those events are natural disasters, disease epidemics, or human wrongdoing.
References
Alexander, M. G., & Fisher, T. D. (2003). Truth and consequences: Using the bogus pipeline to examine sex differences in self-reported sexuality. Journal of Sex Research, 40(1), 27–35. doi:10.1080/00224490309552164
Apfelbaum, B. (1984, November). Sexual reality and how we dismiss it. Paper presented at the meeting of the American Association of the Advancement of Science, San Francisco State University, San Francisco, CA. Retrieved from https://web.archive.org/web/20010627190317/http://www.bapfelbaumphd.com/Sexual_Reality.html
Arfer, K. B. (2016). Diversity of sexual practices is not bad. In Empirical sexual attitudes. Los Angeles, CA: Author. ISBN 978-1-5238-1453-4. Retrieved from http://arfer.net/w/esa/practices
Atkins, D. C., Baucom, D. H., & Jacobson, N. S. (2001). Understanding infidelity: Correlates in a national random sample. Journal of Family Psychology, 15(4), 735–749. doi:10.1037/0893-3200.15.4.735
Biddle, S. (2015, August 19). What's actually inside the nightmare Ashley Madison leak. Gawker. Retrieved from http://gawker.com/1725077740
Blevins, C., & Mullen, L. (2015). Jane, John… Leslie? A historical method for algorithmic gender prediction. Digital Humanities Quarterly, 9(3). Retrieved from http://www.digitalhumanities.org/dhq/vol/9/3/000223/000223.html
Brier, G. W. (1950). Verification of forecasts expressed in terms of probability. Monthly Weather Review, 78(1), 1–3. doi:10.1175/1520-0493(1950)078<0001:VOFEIT>2.0.CO;2
Bröcker, J. (2009). Reliability, sufficiency, and the decomposition of proper scores. Quarterly Journal of the Royal Meteorological Society, 135(643), 1512–1519. doi:10.1002/qj.456
Burdette, A. M., Ellison, C. G., Sherkat, D. E., & Gore, K. A. (2007). Are there religious variations in marital infidelity? Journal of Family Issues, 28(12), 1553–1581. doi:10.1177/0192513X07304269
Buss, D. M., & Shackelford, T. K. (1997). Susceptibility to infidelity in the first year of marriage. Journal of Research in Personality, 31(2), 193–221. doi:10.1006/jrpe.1997.2175
Carroll, J. S., Padilla-Walker, L. M., Nelson, L. J., Olson, C. D., Barry, C. M., & Madsen, S. D. (2008). Generation XXX: Pornography acceptance and use among emerging adults. Journal of Adolescent Research, 23(1), 6–30. doi:10.1177/0743558407306348. Retrieved from https://www.researchgate.net/profile/Laura_Padilla-Walker/publication/247721479/links/00b7d53455d09d6fcd000000.pdf
Coleman, L. M., & Testa, A. (2008). Sexual health knowledge, attitudes and behaviours: Variations among a religiously diverse sample of young people in London, UK. Ethnicity and Health, 13(1), 55–72. doi:10.1080/13557850701803163
Edelman, B. (2009). Red light states: Who buys online adult entertainment? Journal of Economic Perspectives, 23(1), 209–220. doi:10.1257/jep.23.1.209
Feldman, S. S., & Cauffman, E. (1999). Your cheatin' heart: Attitudes, behaviors, and correlates of sexual betrayal in late adolescents. Journal of Research on Adolescence, 9(3), 227–252. doi:10.1207/s15327795jra0903_1
Fisher, T. D., & Brunell, A. B. (2014). A bogus pipeline approach to studying gender differences in cheating behavior. Personality and Individual Differences, 61-62, 91–96. doi:10.1016/j.paid.2014.01.019
Freud, S. (1910). The origin and development of psychoanalysis. American Journal of Psychology, 21(2), 181–218. doi:10.2307/1413001
Fried, J. (2008). Democrats and Republicans—rhetoric and reality: Comparing the voters in statistics and anecdotes. New York, NY: Algora. ISBN 978-0-87586-603-1.
Gallup, G. H., Jr. (2003). Current views on premarital, extramarital sex. Retrieved from http://www.gallup.com/poll/8704/x.aspx
Gelman, A. (2008). Scaling regression inputs by dividing by two standard deviations. Statistics in Medicine, 27(15), 2865–2873. doi:10.1002/sim.3107
Geuss, M. (2016, December 14). Hacked cheating site Ashley Madison will pay $1.6 million to FTC for breach. Ars Technica. Retrieved from https://web.archive.org/web/20161214/https://arstechnica.com/tech-policy/2016/12/hacked
Grieser, W. D., Kapadia, N., Li, R., & Simonov, A. (2016). Fifty shades of corporate culture. Retrieved from http://ssrn.com/abstract=2741049
Griffin, J. M., Kruger, S. A., & Maturana, G. (2016). Do personal ethics influence corporate ethics? Retrieved from http://ssrn.com/abstract=2745062
Hill, T. D., Moulton, B. E., & Burdette, A. M. (2004). Conservative Protestantism and attitudes toward homosexuality: Does political orientation mediate this relationship? Sociological Focus, 37(1), 59–70. doi:10.1080/00380237.2004.10571234
Independence Party of New York. (2014). About/ Legislative Agenda | Independence Party of New York State. Retrieved from http://web.archive.org/web/20141106202740/http://www.nyindependenceparty.com/agenda
Kirby, D. (2007). Abstinence, sex, and STD/HIV education programs for teens: Their impact on sexual behavior, pregnancy, and sexually transmitted disease. Annual Review of Sex Research, 18(1), 143–177. doi:10.1080/10532528.2007.10559850
Lamont, T. (2016, February 27). Life after the Ashley Madison affair. The Guardian. Retrieved from https://www.theguardian.com/technology/2016/feb/28/what-happened-after-ashley-madison-was-hacked
MacInnis, C. C., & Hodson, G. (2015). Do American states with more religious or conservative populations search more for sexual content on Google? Archives of Sexual Behavior, 44(1), 137–147. doi:10.1007/s10508-014-0361-8
McArdle, J. (2007, August 27). Craig arrested, pleads guilty following incident in airport restroom. Roll Call. Retrieved from http://web.archive.org/web/20150908081135/http://www.rollcall.com/news/-19763-1.html
Moe, K. (1984). Should the Nazi research data be cited? Hastings Center Report, 14(6), 5–7. doi:10.2307/3561733
Paton, C. (2015, August 24). Ashley Madison hack list: How to download and search leaked adultery website database email addresses. International Business Times. Retrieved from http://www.ibtimes.co.uk/1516808
Petersen, L. R., & Donnenwerth, G. V. (1997). Secularization and the influence of religion on beliefs about premarital sex. Social Forces, 75(3), 1071–1088. doi:10.2307/2580531
Pew Research Center. (2009). Trends in political values and core attitudes: 1987-2009. Author. Retrieved from http://www.people-press.org/2009/05/21/independents-take-center-stage-in-obama-era
Pew Research Center. (2015). A deep dive into party affiliation: Sharp differences by race, gender, generation, education. Retrieved from http://www.gallup.com/poll/8704/x.aspx
Prince, S. J. (2015, August 19). Where can I find Ashley Madison data? Heavy. Retrieved from http://heavy.com/news/2015/08/how-to-download-ashley-madison-full-email-list-dump-torrent
Sidanius, J. (1985). Cognitive functioning and sociopolitical ideology revisited. Political Psychology, 6(4), 637–661. doi:10.2307/3791021
Stanger-Hall, K. F., & Hall, D. W. (2011). Abstinence-only education and teen pregnancy rates: Why we need comprehensive sex education in the U.S. PLOS ONE. doi:10.1371/journal.pone.0024658
Steel, E., & Schmidt, M. S. (2017, April 19). Bill O'Reilly is forced out at Fox News. The New York Times. Retrieved from https://www.nytimes.com/2017/04/19/business/media/bill-oreilly-fox-news-allegations.html
Strang, E., & Peterson, Z. D. (2016). Use of a bogus pipeline to detect men's underreporting of sexually aggressive behavior. Journal of Interpersonal Violence. Advance online publication. doi:10.1177/0886260516681157
Tuttle, J. D., & Davis, S. N. (2015). Religion, infidelity, and divorce: Reexamining the effect of religious behavior on divorce among long-married couples. Journal of Divorce and Remarriage, 56(6), 475–489. doi:10.1080/10502556.2015.1058660
United States Census Bureau. (2012). Table 1. Annual estimates of the population for the United States, regions, states, and Puerto Rico: April 1, 2010 to July 1, 2012 (NST-EST2012-01). Retrieved from https://www2.census.gov/programs-surveys/popest/tables/2010-2012/state/totals/nst-est2012-01.xls
Victor, D. (2015, August 19). The Ashley Madison data dump, explained. The New York Times. Retrieved from http://www.nytimes.com/2015/08/20/technology/the-ashley-madison-data-dump-explained.html
Whitley, B. E., Jr., & Lee, S. E. (2000). The relationship of authoritarianism and related constructs to attitudes toward homosexuality. Journal of Applied Social Psychology, 30(1), 144–170. doi:10.1111/j.1559-1816.2000.tb02309.x
Yoon, H.-J., Tourassi, G., & Xu, S. (2015). Residential mobility and lung cancer risk: Data-driven exploration using internet sources. Lecture Notes in Computer Science, 9021, 464–469. doi:10.1007/978-3-319-16268-3_60. Retrieved from http://www.ncbi.nlm.nih.gov/pmc/articles/PMC4662563/
Zabin, L. S., Hirsch, M. B., Smith, E. A., & Hardy, J. B. (1984). Adolescent sexual attitudes and behavior: Are they consistent? Family Planning Perspectives, 16(4), 181–185. doi:10.2307/2134899